For his work “Machine learning assisted signal analysis in Acoustic Microscopy for non-destructive defect identification” Michael Kögel from the Fraunhofer Institute for the Microstructure of Materials and Systems IMWS in Halle (Saale) received the prize for the “Attendees‘ Best Paper” awarded by the international symposium ISTFA 2019 in Portland/USA.
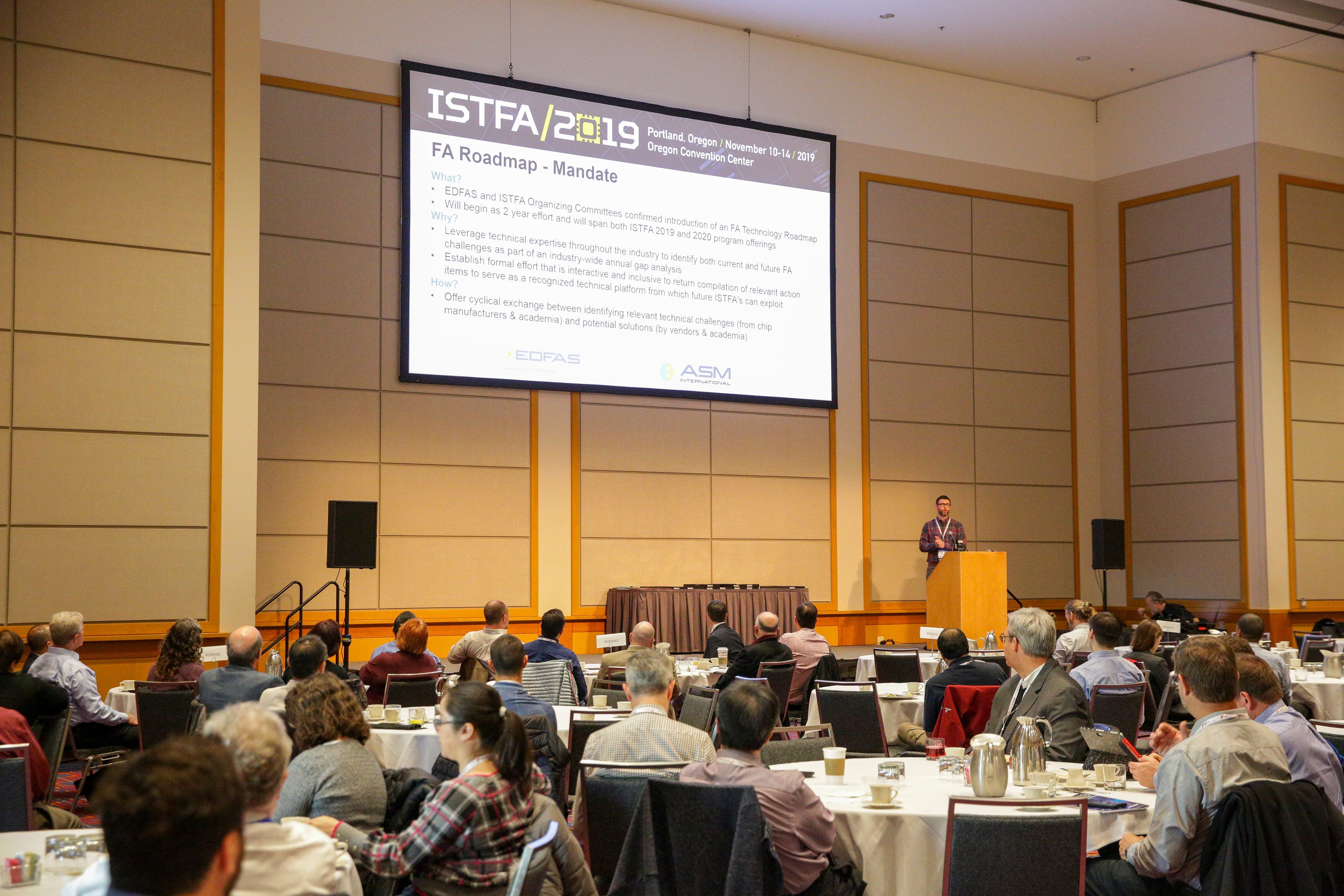
The “International Symposium for Testing and Failure Analysis (ISTFA)” is one of the world’s most important symposia for failure analysis in materials sciences. The Fraunhofer IMWS is a regular attendee and presents new findings centering on error diagnostics and analysis of microelectronic technologies.
The work published by Michael Kögel with the collaboration of Dr. Sebastian Brand and Frank Altmann from the Fraunhofer IMWS illustrates the opportunities of combining two innovative technologies: acoustic microscopy (SAM, Scanning Acoustic Microscopy), i.e. the study of materials using focused ultrasound, enables the non-destructive search for errors in substances and complex systems. Machine learning can be used to analyze the signals measured more quickly and reliably and extract relevant findings from the data.
“Signal processing and data interpretation using acoustic microscopes is often challenging and is based on subjective decisions taken by the operator. The results in terms of how the defect is recognized and classified rely to a large extent on experience. We have developed a method expanding the possibilities currently available when a signal is analyzed, enabling the operator to take independent, objective decisions based on the measurement data”, says Kögel.
In the paper the experts from the Fraunhofer IMWS described two methods which use and also combine supervised and unsupervised learning for extracting properties and image segmentation. This enables automatic classification and predictive error analysis based on the SAM data.
For example the researchers studied a defective CPU (Central Processing Unit) assembled using flip chip technology. In this method, used primarily in extremely complex circuits, the chip’s active contact side is directly connected to the substrate, i.e. without any need to use wires to create the electrical contacts. The contact points between the substrate and the chip’s circuit structure are called bumps and are created using coatings and etching techniques. Defects can arise during this process – defective bumps break the electrical connections in the circuit and have a resulting impact on functionalities. Information about such defects can be collected in a non-destructive manner using an acoustic microscope. However, the information may be “concealed” in the echo signals measured.
The approach developed by Kögen and his colleagues employs a large quantity of data and identifies any conspicuous signal components of the acoustic echo signals, thus enabling conclusions to be drawn about the condition of the bumps. With regard to whether accurate predictions can be made using machine learning, the Fraunhofer researchers examined the chips in question using high-resolution imaging techniques and were able to verify their model.
Using further data, the model, based on a one-dimensional Convolutional Neural Network (CNN), can be further trained for fine adjustments. “The model was sensitive enough to pick up critical properties of the acoustic echo signals and enabled the automatic classification of intact bumps, defective bumps and separate sample structures. We also managed to validate this approach through an additional physical analysis of the test samples, which contained several flip chip contact defects”, explains Kögel. The classification model attained a degree of accuracy of over 97% and has already been successfully applied to an unknown sample. Kögel is convinced of the potential of the concept of machine learning: “In the future artificial intelligence will be able to help us make error analysis more efficient and precise.”